
Why GPT4 Isn’t Ready For Data Insight Primetime (yet).
Why GPT4 Isn’t Ready For Data Insight Primetime (yet). https://www.citizenme.com/wp-content/uploads/2023/04/deepmind-AI-Paths-unsplash.jpeg 7500 4500 StJohn Deakins StJohn Deakins https://secure.gravatar.com/avatar/67e7ca4885d1b922783ca3a83741a282?s=96&d=mm&r=gOK, so firstly, don’t get me wrong on the title. If you’re hoping for a luddite rant, this isn’t it!
The latest large language model (LLM) Generative AI’s such as GPT4 from OpenAI are a phenomenal advance. The rapidly increasing number of other models arriving for the masses from Google, Meta and many others are awesome too. They will undoubtedly have a huge impact on the personal data Insights industry and there are some awesome tools emerging.
At CitizenMe, our team is thrilled about the major advancements in AI such as OpenAI’s GPT4. We’re currently developing other flavours of AI that we call ‘edge AI’, designed to improve people’s lives in a private manner. We call these ‘edge AI’ because they’re integrated into personal CitizenMe apps and operate on locally collected and stored 360º life data. In the data marketing industry, this approach is referred to as ‘Zero-Party Data’ (ZPD), and we are also creating ‘Zero-Party AI’ through this approach. By validating and analysing the data at the source, our technology can provide businesses and organisations with more accurate, comprehensive, safe, and ethical human data insights.
And there is the rub for GPT4 and the current crop of Large Language Models (LLM’s). They’re simply not ready for us to use, just yet.
Our team has been testing some LLM models and analysing their outputs. Our goal is to include them in our upcoming Data Science releases in 2023. However, we don’t think these models are ready to be used for analysing anonymous survey data, let alone for analysing live data with personally identifiable information (PII) to give individuals personal insights. There are two main concerns with using this type of data science in its current state:
1) The General Set up of Today’s Early LLM’s:
Transparency & Ethics
GPT specifically has been trained on the personal data of millions of people, it’s probably actually hundreds of millions, and it’s possibly been trained on data of a billion or more people – and that’s the issue, we simply don’t know.
- Web pages and articles: Web pages and articles from the internet. This likely includes PII, but no one can be sure, including OpenAI.
- Books: huge back catalogues of digitised fiction and non-fiction books.
- Social media posts: data such as tweets, posts, and comments from platforms like Twitter, Reddit, and Facebook.
- News articles: News articles from various sources and languages. The provenance (bias) of the news sources is not clear.
- Scientific articles: Used to train the model on technical language and domain-specific knowledge. These may inadvertantly include PII and PHI.
- Conversational data: Chat logs and dialogues (with humans), from a wide variety of sources to help generate more naturally sounding responses.
The precise training data used, is an OpenAI company secret. How this impacts new personal data rights such as the right to be forgotten under GDPR is not clear.
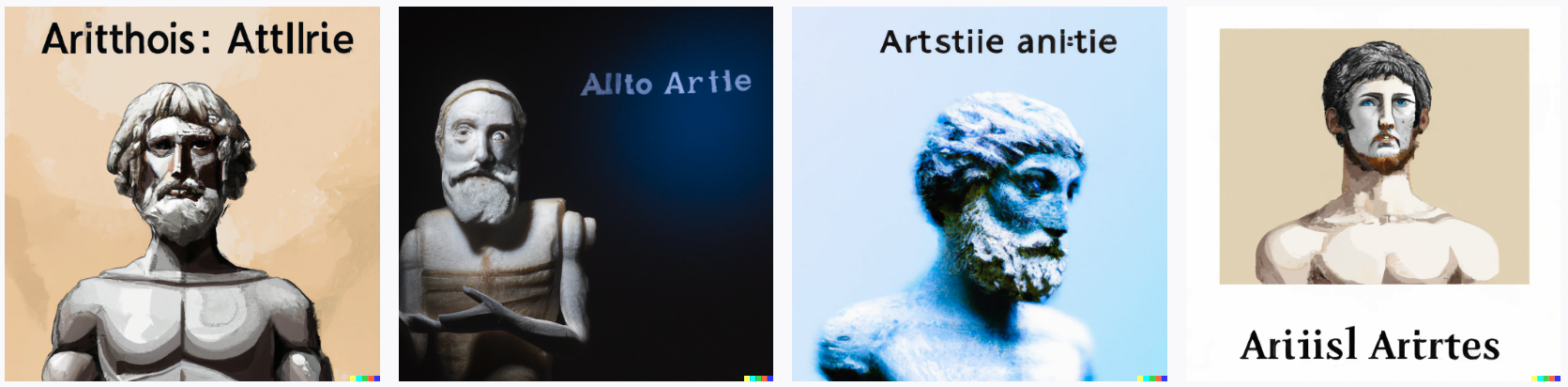
Source: DALL.E Prompt: Aristotle as an AI
Privacy & Regulatory
For us humans, this means that peoples data has been scraped from around the internet without any form of consent. There is very little in the way of privacy protection and there is no way of knowing if your data has been sucked into the model.
For this reason, in Italy OpenAI’s Chat GPT and GPT4 have been banned by the data regulator. The Italian regulator has laid out it’s requirements including giving Italian citizens a ‘right to have their data *and* any incorrect or ‘hallucinated’ results (more below) must be removed from the output. Given the structure of the system, and the purported 10 trillion ‘parameters’ used, this may be much easier said than done.

Source: DALL.E Prompt: AI Digital Privacy and Regulation
Security
For the new entrants like OpenAI, their security systems are “beta” at best with a number personal data leaks coming to light in recent months. Most issues to date have been in the configuration of systems and code libraries. In many other industries there is a high bar for reliability. For example, health data is protected by GDPR and HIPAA in the EU and USA respectively. Similarly, Telecoms companies stipulate that systems must be 5X9’s reliable (as in they must be up and available 99.999% of the time). For the moment at least, most LLM/GPT AI systems are primarily marketed as ‘beta’ and ‘research’ services with no significant guarantees to users on security, risk mitigation and service availability.

Source: DALL.E Prompt: AI Cyber Security
Confidentiality
AI models use all the prompts and outputs made to further train their models. With a centralised Large Language Model AI, it is currently impossible to limit certain documents to specific users or groups. This means that any confidential information fed into the model as a prompt becomes part of the larger model, and potentially searchable by all other users.
This is awesome for creating collective *open knowledge* within a community – but less so if it means sharing private and confidential information with others in sensitive environments (including competitors in a business) or sensitive PII in a health environment. Samsung recently discovered that their engineers have been using generative AI models to build and optimise their code – all of which is now sitting in publicly available (and privately) owned LLM libraries. OpenAI promise in their T’s & C’s that purchasers of ‘enterprise’ API access also purchase confidentiality of the data shared with the model. However, given the data opacity and security glitches already happening (see above), we’re not quite ready to trust them on this just yet.
2) The Output
It Get things wrong
AI’s are still art an early stage, they get things wrong – For example when Google launched it’s Bard LLM-AI and got our understanding of the universe muddled up.
Similar to linear regression, the overall picture may be convincingly generally correct (and very convincingly so), however the individual data points will often be wrong (azeem Azar pic)
In our experiments with AI we’ve found this to be true…
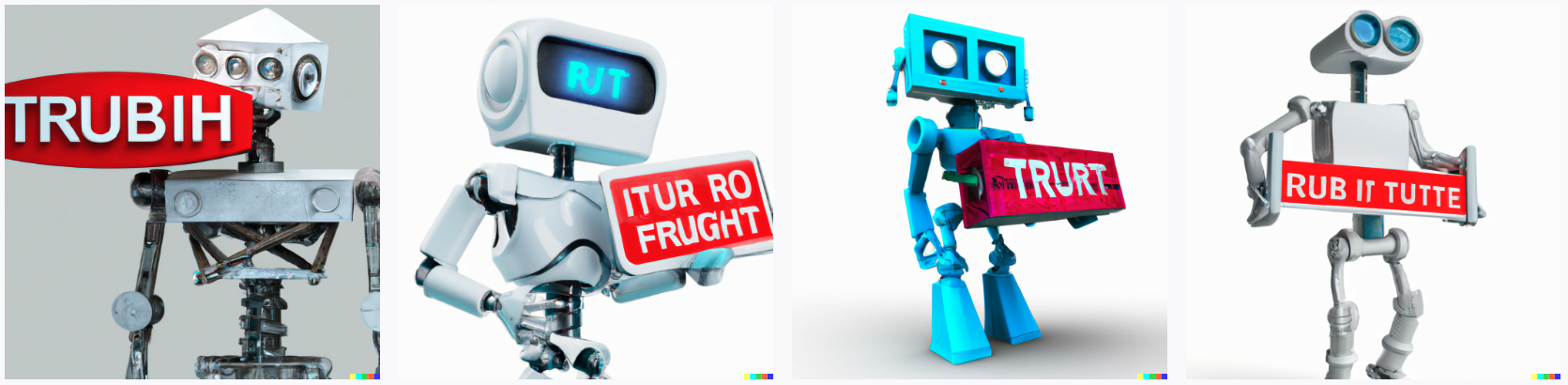
source: DALL.E Prompt: untruthful AI robot
It makes stuff up with ‘heuristic hallucinations’
Worse still, if LLMs aren’t sure of an answer, they will take a guess and ‘hallucinate’. In short LLMs make stuff up. It’s a quirky mirror of human heuristics, where people might use biases and mental shortcuts to get to a broadly correct answer. When humans do it, it’s either an educated guess, or bullshit. When LLMs do it, they can be very convincing at sounding authoritative, but wrong. Sometimes it’s humorous, but sometimes is defamatory and potentially life damaging. So LLM’s and Generative AI is ready for mis-truth, but is it ready to elevate truth?

Source: DALL.E Prompt: “hallucinating GPT dreams”
Where we are at:
The space is moving very quickly. For example Databricks has just announced an open source AI for commercial (not just research) use that it claims is as good as ChatGPT *and* uses ‘ethical’ training parameters. We expect that these advances in the models, and their efficiency, will continue to accelerate.
For now, we are continuing with the deployment of our own edge AI programme, starting with in-app recommenders and followed by data veracity scores. Any AI is only as good as the strength of truth in its source data.
Transparency about the provenance and veracity of data is essential. Doing this in a ‘human first’ way, with ethics baked in, is foundational. Enabling people to participate with their own data in these models will be transformational.
In the medium term we’re experimenting with creating “edge AI” LLM’s in personal data apps with thousands of private and secure 360º life data points as an ethical and human owned ‘personal AI’.
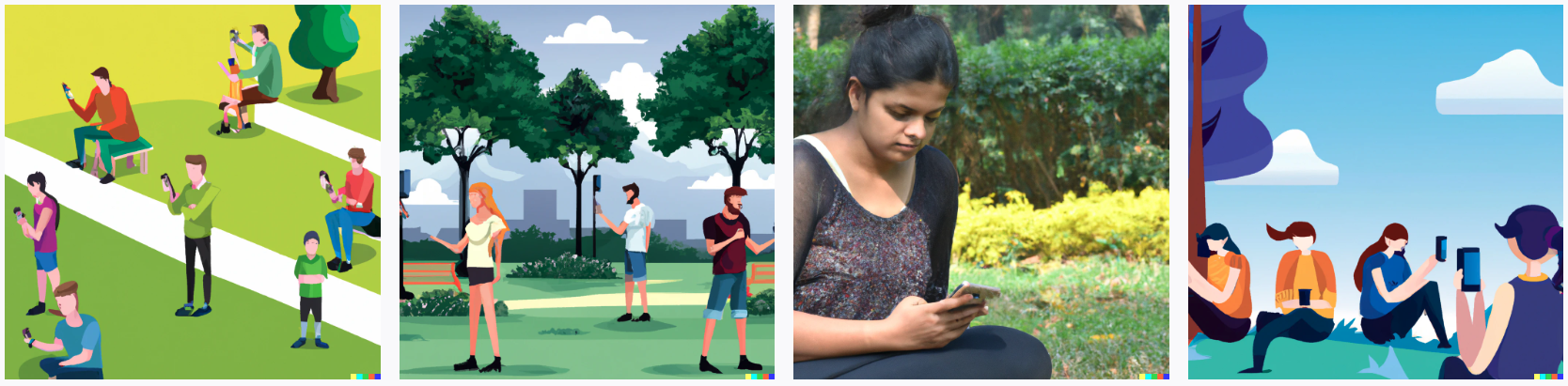
Source: DALL.E Prompt: humans in a park with smartphones
Conclusion:
In an industry that seeks truth from data; that prides itself on calculating accurate incidence rates; that expects disclosure of margins of error as standard; and couches recommendations with caveats and counter-points… We need better. Yes GPT4 and other LLMs are wonderful and promise exciting efficiencies. However, we need advances in Human Data AI that also galvanise ethics and morals. Perhaps above all in our ‘post-truth world, we need AI that advances ‘truth’.
Our team is certain that LLM’s and Generative AI will revolutionise many industries, including Consumer Insights – and possibly more quickly than anticipated. However, we need to advance with our eyes wide open to the realities as we bridge the phase transition.
In Q2 2023 we’re launching an Open 360 Life Data Lab to explore this new frontier openly and collaboratively. Get in contact if you’d like to get involved, or find out more (Human Intelligence included 😉).
- Post Tags:
- #Data Science
- 360º life data
- AI
- Chat GPT
- Citizen Data Lab
- CitizenMe
- data insights
- data veracity
- data veracity scores
- Edge AI
- Ethical Data
- GDPR
- Generative AI
- GPT
- GPT4
- heuristic hallucinations
- HIPAA
- Human Data
- Large Language Models
- life data
- LLM
- Marketplace
- Open 360 Life Data Lab
- open knowledge
- OpenAI
- personal AI
- personal health information
- personally identifiable information
- PHI
- PII
- Zero Party Data
- Zero-Party AI
- ZPD
- Posted In:
- Uncategorized
StJohn Deakins
StJohn founded CitizenMe with the aim to take on the biggest challenge in the Information Age: helping digital citizens gain control of their digital identity. Personal data has meaning and value to everyone, but there is an absence of digital tools to help people realise its value. With CitizenMe, StJohn aims to fix that. With a depth of experience digitising and mobilising businesses, StJohn aims for positive change in the personal information economy. Oh… and he loves liquorice.
All stories by: StJohn Deakins